6 min read
The CIO's role in shaping the AI Future
Listen to this blog post instead:
The CIO's role in shaping the AI Future
CIOs are already pioneers, strategists and mentors for their organisations. They don’t necessarily get involved in the actual delivery of projects and innovation. But the unchartered territory of AI changes this dynamic slightly.
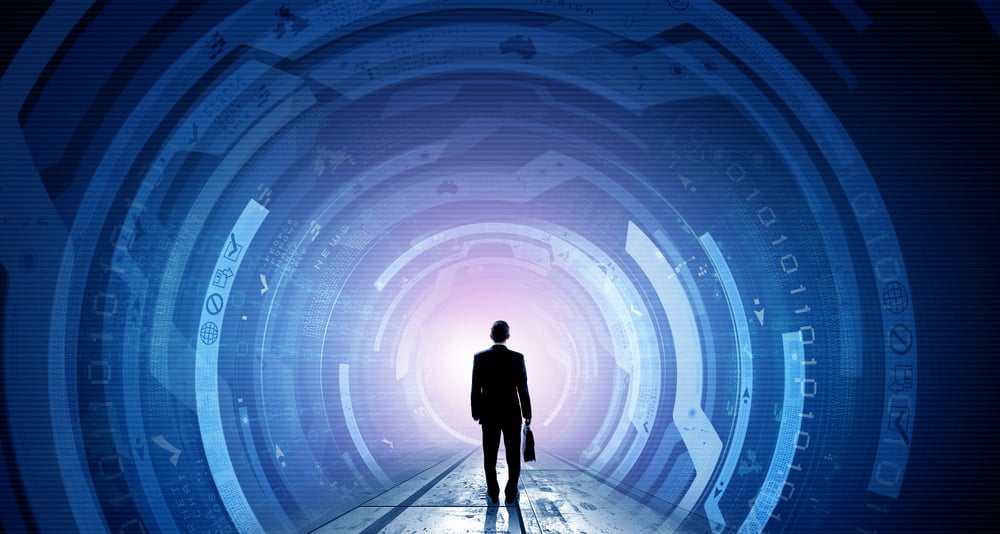
When it comes to AI programmes of work, beyond strategic navigation the role of the CIO widens slightly to have a greater presence in iterating and delivering this revolutionary technology.
Bringing this all to life is managing digital budgets, ensuring multi-disciplinary projects and initiatives are scoped and funded appropriately. AI and data investments are likely to take an increasing share of the cake over the next few years, as confirmed in research by the MIT Technology Review and Foundry.
Leading from the front as a key AI collaborator
In many ways, AI continues to be a novel technology with ground-breaking features and use cases that are continuously being developed. Inspired by AI, CIOs need to consider how to be a digital business enabler, not just a technology provider.
Put simply: CIOs have the exciting opportunity to both lead from the front line – but also become a key collaborator in pioneering and molding the capability of AI for their organisation. To discover this capability, our white paper reveals how CIOs should experiment with AI wisely in a digital world where there is limited direction or unproven legacy.
Experienced AI practitioners are highly specialised. But that doesn’t necessarily mean having to look far and wide.
Successful AI delivery requires multiple roles with differing skillsets, so assembling the right team is often the key component to a great delivery. An effective enterprise deployment of AI requires well-rounded, blended teams that draw on people from diverse backgrounds and skill sets, including non-technical roles.
This team, for example, might include:
- Domain experts and people who understand business processes. They can deliver a product that aligns to company goals and be able to articulate what good looks like.
- Experienced analytics professionals who understand machine learning, statistics, forecasting or optimisation. They’ll help you to make sense of your data and will help guide the best techniques.
- People who are knowledgeable about data origins, its quality, and know how to handle it properly.
- Strong software developers who are skilled in delivering enterprise-grade software solutions. Architects with a strong vision of turning new technology concepts into operational realities.
- Trusted professional services organisations that can advise on best practice, product selection, and handle technical implementation if required – from concept to completion.
Assembling such a broad multi-skilled team might be easier said than done. The CIO must consider several factors about each person in the team, including experience, education, skillsets, extent of influence and their commitment to delivery.
Once this symphony of professionals to research, deliver and execute new AI technologies and methodologies is assembled, the CIO should then set the team up for success.
Implementing your AI Engineering/MLOps
This fairly novel DevOps-inspired approach involves orchestrating a holistic integration of people, processes and technologies. At its core, this strategy creates an environment where AI projects can thrive, from conception to deployment. The first step is to establish a centralised AI Engineering Platform, a dynamic space where data scientists, engineers, and domain experts converge.
This platform acts as a nexus, encouraging collaboration, enabling version control, and promoting the reuse of successful models. By providing a unified space for experimentation and model training, organisations can significantly reduce the time from ideation to prototype, empowering teams to iterate swiftly and innovate effectively.
In parallel, a robust governance model acts as the guiding beacon for AI initiatives. As a CIO, you must:
- Outline clear policies regarding data usage, model ethics, and compliance standards
- Define roles and responsibilities within AI teams, ensuring accountability and clarity
- Ensure proper governance so that AI projects align with organisational objectives, adhere to regulatory requirements, and uphold ethical considerations.
This structured approach enhances both the credibility of AI solutions and instils confidence among stakeholders, paving the way for responsible AI implementation.
The incorporation of DevOps principles into AI development, encapsulated within the concept of MLOps, marks another crucial milestone. MLOps bridges the gap between machine learning development and digital operations, enabling seamless collaboration between data scientists and digital operation professionals.
Define Objectives:
Begin by clearly defining the objectives and goals ahead of the AI pipeline. Understand what problem must be solved, what data is needed, and what outcomes are expected.
Data Collection:
Collect and gather the data required for the AI project. Ensure the data is relevant, accurate and properly categorised.
Building your consistent AI Pipeline
Interoperability between data scientists and developers
This process involves meticulously architecting the flow of data, algorithms and insights, ensuring a seamless journey from conception to implementation. The first step to this is standardisation. CIOs must standardise tools, frameworks and libraries across all AI projects. This uniformity ensures:
- Interoperability, easing the collaboration between data scientists and developers.
- The rapid onboarding of new team members, working within a familiar digital and delivery landscape, enhancing productivity and knowledge sharing
Another vital aspect of the AI pipeline architecture is continuous validation and testing. In the realm of AI, where complex algorithms can often lead to unpredictable outcomes, rigorous testing is indispensable. CIOs should invest in robust testing frameworks that encompass both unit testing of individual components and integration testing of the entire system.
Importantly, implementing automated testing protocols quickly identifies anomalies, enabling teams to quickly iterate and refine their models. Regular validation ensures AI solutions are both accurate during development, but also resilient in real-world scenarios, bolstering confidence in their application.
Leveraging Cloud-Based AI Platforms
Cloud infrastructures such as AWS, with its inherent flexibility and scalability, have reshaped how organisations harness the power of artificial intelligence. CIOs navigating the complexities of AI implementation increasingly turn to cloud-based solutions due to their proven advantages.
One of the primary benefits lies in the ability to start small Proof of Concepts (POCs) and experiment in a demand-driven manner. Cloud platforms enable businesses to allocate resources judiciously, focusing precisely on building models and achieving swift victories. This 'experiment, pilot, learn and scale' approach both mitigates the risks attached to large-scale investments and ensures resources are channelled into activities that yield tangible results. Organisations can focus on achieving specific business outcomes, abstracting away the technological intricacies that often hinder early stages of AI adoption. This streamlined approach both optimises costs but also expedites the path from proof of concept to real-world applications.
Leveraging diverse services
Flexibly, cloud-based AI platforms empower organisations to tap into a vast array of AI capabilities. The versatility offered by the cloud enables enterprises to leverage diverse services, from Machine Learning (ML) algorithms to natural language processing tools, catering for a wide spectrum of applications.
Businesses can experiment with cutting-edge AI technologies without the burden of hefty upfront investments in specialised hardware or software. This accessibility democratises innovation, allowing both startups and established enterprises to explore the full potential of AI without prohibitive barriers.
The cloud’s dynamic scalability aligns seamlessly with the evolving nature of AI workloads. As the demand for AI services fluctuates, cloud resources can be effortlessly scaled up or down, ensuring optimal performance during peak times while optimising costs during periods of lower activity. This adaptability guarantees that organisations respond swiftly to market demands and evolving customer expectations, enhancing their competitive edge in the digital arena.
Unite teams through shared environments
Moreover, cloud-based AI platforms drive collaboration and knowledge exchange within organisations. These platforms converge cross-functional teams, from data scientists to developers, by providing shared environments for experimentation and development. Real-time collaboration becomes not just a possibility but a reality, enabling teams to collaborate seamlessly on projects, iterate on models, and troubleshoot challenges collectively.
The cloud’s collaborative tools and integrated development environments enhance communication and foster a culture of innovation. Additionally, cloud providers often offer extensive libraries, frameworks, and pre-trained models that expedite development cycles. This wealth of resources empowers developers and data scientists to focus on refining AI models and driving business value rather than reinventing the wheel. By promoting collaboration and knowledge sharing, cloud-based AI platforms nurture an environment where ideas flourish.
And what can this ultimately achieve? Groundbreaking innovations that define the future of industries.
By embracing the cloud, CIOs position their organisations at the forefront of innovation, equipped to harness the true potential of AI.
Implementing a Governance Model for AI
Define principles that set ethical boundaries
In the rapidly evolving AI space, where data-driven decisions permeate every aspect of operations, a Governance Framework becomes indispensable. The first critical component of this model is the establishment of clear ethical guidelines. CIOs, in collaboration with their legal and operational experts, must define principles that spell out the ethical boundaries in which the AI systems will operate. These principles cover issues such as:
-
Bias mitigation
-
Privacy preservation
-
Transparency
Data management forms another crucial dimension of the governance model. It defines protocols for data collection, storage and usage. CIOs spearhead the creation of policies that ensure data integrity, confidentiality and security. Rigorous data governance measures are essential to maintain the quality of input data, as AI systems rely heavily on the accuracy and relevance of the data they’re trained on. Additionally, these policies specify the permissible uses of data, preventing unauthorised access and ensuring compliance with legislation.
Importantly, a governance model must address the interpretability and accountability of AI systems. Transparency mechanisms are integrated into AI algorithms, enabling stakeholders to understand the rationale behind AI-driven decisions. Additionally, accountability measures, such as audit trails and documentation, are put in place to trace the lineage of AI decisions.
These measures enable organisations to identify and rectify errors or unwanted practices, ensuring AI systems remain accountable and aligned to the organisation’s values. By establishing a comprehensive governance framework encompassing ethics, data management, interpretability, and accountability, CIOs can empower their organisations to remain innovative, but in a responsible manner.
Conclusion
In the realm of AI MLOps engineering, AI pipelines, cloud-based AI platforms, and the critical aspect of governance models for AI, the CIO's role shifts slightly. The convergence of these domains represents a profound shift in the CIO's ability to drive innovation, enhance operational efficiency, and deliver transformative solutions.
The role of the CIO is to steer the organisations through the complexities of this rapidly evolving – and in some cases, untested - technology and drive data-driven decision-making. AI takes their existing qualities of pioneers, strategists, and ethical compass of the organisation, and adds to it by allowing the CIO to drive and deliver from the trenches.
Ultimately, AI offers unprecedented opportunities do drive innovation, but where do you start as a pioneer on your journey when experimenting with its vast capabilities?
Our white paper provides strategic guidance and valuable insight on how to achieve this.